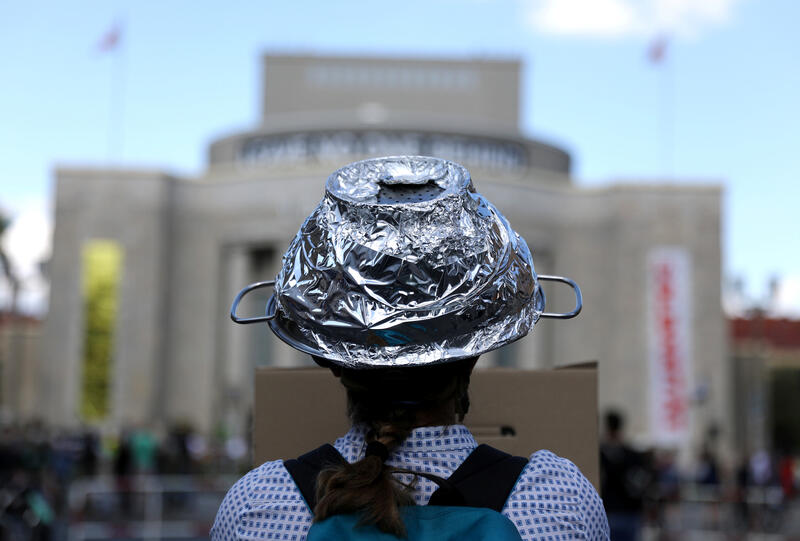
A woman attends a protest of conspiracy theorists and other demonstrators at Rosa Luxemburg Platz, amid the spread of the coronavirus disease (COVID-19), in Berlin, Germany May 9, 2020. REUTERS/Christian Mang
A woman attends a protest of conspiracy theorists and other demonstrators at Rosa Luxemburg Platz, amid the spread of the coronavirus disease (COVID-19), in Berlin, Germany May 9, 2020. REUTERS/Christian Mang
In this RISJ Factsheet, we assess the volume and patterns of toxic conversations on social media during the COVID-19 pandemic. We specifically analyse worldwide conversations on Twitter targeting the World Health Organization (WHO), a central actor during the pandemic. Our analysis contributes to the current research on the health of online debates amid the increasing role of social media as a critical entrance to information and mediator of public opinion building.
Following previous studies on the field, for this analysis we define toxicity as ‘a rude, disrespectful, or unreasonable comment that is likely to make people leave a discussion’ (Wulczyn et al. 2017). Consistently, we identify the probability that a tweet conveys a toxic message and then calculate the overall volume of toxicity on Twitter across time. Then, we identify the contextual information and external events that potentially help to understand when and how toxic messages gain momentum on social media during the COVID-19 pandemic. Finally, we explore potential coordination dynamics behind the spread of those messages, identifying parallel campaigns of toxic messages targeting a narrow set of countries or other global actors.
Our analyses are based on a filtered dataset of about 303 million tweets including COVID-19 related terms, from which we obtained a final sub-subset of 222,774 tweets mentioning the WHO. The time window for this study spans 20 January to 23 April 2020. At that time, countries were at different stages of the pandemic. Some of them – mainly European, but also others such as China – were facing the most severe consequences of the peak of the outbreak, including strict lockdown measures, whereas others were just through the first stages of the crisis (see Figure 1 for more contextual information).
Figure 1. Timeline of selected COVID-19 events
Note: Selection of COVID-19-related worldwide events. The size of the bubbles represents the number of world COVID-19 cases confirmed. Own elaboration based on data from Johns Hopkins University and Medicine (2020).
Toxic messages amount to 21% of the overall conversation touching on the COVID-19 pandemic and the role of the WHO in the crisis. In other words, 21 out of 100 tweets in our sample are expected to convey a rude, disrespectful, or unreasonable comment.
The percentage of toxic tweets increases after 26 March (25%), when many countries were facing the growing adverse effects of the pandemic and passing measures to confine their populations.
Peaks in toxicity can be divided into two different phases. At the beginning of the pandemic the highest percentage of toxic messages correlate with the WHO’s statements or events, whereas at the end of the period studied, top-down messages from political leaders or specific media coverage coincide in time with the surge in toxicity.
Coordinated efforts to boost toxicity are detected after 26 March, when a set of hashtags targeting the WHO simultaneously emerge conveying messages with a higher average toxicity. Up to 33% of the total conversation including those hashtags was toxic. Evidence shows this conversation was correlated with several parallel campaigns of toxic messages targeting a narrow set of other actors and topics at the same time, including China, Taiwan, the idea of COVID-19 as a Chinese virus, or the conspiracy theory associating it with a biochemical weapon.
A higher level of toxicity than the average baseline is detected in conversations around political leaders. Notably, among those, over 30% of messages mentioning the US president are expected to be toxic.
Five months after the beginning of the outbreak, around 7% of accounts that participated by posting or retweeting messages about COVID-19 and the WHO were not active, i.e. either suspended or not found. The percentage of non-active users is over 10% for specific hashtags with higher toxicity scores on average, such as #WHOLiedPeopleDied.
Measuring toxicity is a proxy to assess the civility of the online debate. Civil conversations are considered a cornerstone of democracy, in part because the lack of these has a negative impact on trust in the political process (Mutz 2015). Evidence is not conclusive, and some argue that certain instances of incivility can serve democratic ends, such as encouraging people to engage in tough conversations (Sydnor 2019). Yet, incivility has been broadly deemed to hurt citizens’ involvement in reasoned discussions and increase extreme political polarisation (Stryker et al. 2016). The increasing incivility of social media conversations currently dominates expert and policy debates, and the academic research too (Alba et al. 2020; European Commission 2020; House of Lords 2020; Kosmidis and Theocharis 2019; Zuckerberg 2020).
Social media are considered by some as part of the institutional underpinnings of democracy (Margetts 2017). Against this backdrop, what happens on platforms such as Twitter has effects beyond the digital sphere. Remarkable examples of this are found in countries where social media have been used to distract public opinion, to limit collective action, or to stifle dissent (Facebook 2018; King et al., 2017; Tucker et al. 2017). Problems associated with social media are, therefore, important for democracy.
We focus our study on one of these problems, i.e. toxic conversations during the COVID-19 pandemic. More specifically, we look at Twitter for various reasons. The percentage of Twitter users worldwide varies across countries, and in some of them it might look negligible when compared to that of Facebook. The latter is the most used social media platform for any purpose. On average, 63% of people use Facebook across 12 countries, including the UK, the US, Japan, Brazil, and several EU countries. Only around 23% of the population of those countries uses Twitter (Newman et al. 2020).1
Among those who use Twitter the most are political elites, journalists, and other influential actors, such as celebrities. All of them have the potential to spur global attention (Gonzalez-Bailon et al. 2013). Adding to this, to understand the importance of the platform beyond usage figures, journalists give a substantial amount of attention to the activity on Twitter, and some even deem information circulating there more newsworthy than that from established news sources (McGregor and Molyneux 2020). By picking up stories on Twitter, journalists amplify its reach among offline audiences too. The COVID-19 pandemic is replete with examples of messages that quickly jumped over the boundaries of the Twittersphere and became a global debate after being mediated by mainstream outlets (among the prominent ones see Scott 2020; Trump 2020; Zhao 2020).
Global and local conversations related to the COVID-19 pandemic on Twitter occurred alongside an increasing volume of uncivil conversations. A significant amount of those conversations targeted the WHO, one of the global actors in this crisis. Interestingly, in late March and early April this year, people surveyed across several countries, including Argentina, the UK, and South Korea, expressed very high levels of trust in global health organisations such as the WHO. Around that time, 68% of the US public at large rated these organisations as trustworthy – although only 51% of Americans on the political right did so (Nielsen et al. 2020). The WHO was also linked and promoted by platforms as a credible source of information in its various attempts to combat misinformation (Twitter 2020a). Yet, as the following sections will show, its broadly uncontroversial expertise, as measured by high approval ratings across countries, contrasts with the volume of uncivil conversations targeting the organisation at the same time on social media. To understand the nature of this phenomenon and how it evolved across time, we proxy uncivil conversations by specifically analysing the toxicity that emerged from those messages.
Data for this study are obtained from a bigger dataset including 303 million tweets and collected via the Twitter Streaming API from 20 January to 23 April. During that time period, tweets including #COVID-19 and related terms2 were gathered without language or geographical limits.3 The volume of daily tweets posted surged after early March, when peaks of 4.5 million tweets were registered on several days as more countries were affected by the COVID-19 outbreak and its consequences for public health worsened in others.
For our analyses, we filtered the raw dataset to get only those messages mentioning the WHO and related officials of the organisation.4 The resulting sample includes 222,774 tweets (70,966 unique tweets). To identify toxicity of messages in our dataset, we rely on a machine learning approach. We use a classifier already trained to indicate the probability that a text resembles patterns of a toxic message (Jigsaw 2017; Wulczyn et al. 2017). This model returns a score ranging from 0 to 1 associated with each message.5 Higher numbers represent a higher likelihood that a text is considered toxic6 (for more on this model see Jigsaw 2017; Wulczyn et al. 2017). As an example of these processes, see below the scores obtained for tweets by two different public figures:7
You lazy, [obscenity]. You knew TWO MONTHS AGO there would be a #COVID19 Pandemic & you chose not to act. Then u lied about it. You [obscenity]. People have died that wouldn’t have if you’d only done your goddamn job. [obscenity] you forever @realDonaldTrump You sad, [obscenity] (@otepofficial on Twitter, 2 April. Singer. Score=0.97)
Herd Immunity- some voices -'It’s dangerous strategy' - Devi Sridhar global public health Edinburgh Univ -'They are alone in world. It’s a gamble' - Peter Drobac global health, infectious disease Oxford Univ -'The greatest error is not to move' - Dr Mike Ryan WHO #COVID19 (@paul__johnson on Twitter, 14 March. Journalist. Score=0.12)
During the period of study, 2,370 tweets about COVID-19 and the WHO were posted daily on average, including retweets (Table 1). The highest volume of tweets on a single day (n=11,482) was recorded on 15 April, one day after the US confirmed it was going to halt funds to the WHO and the Director-General of the organisation publicly responded to that plan. As we will show in the following section, we identify surges in toxic conversations coinciding with this peak.
Table 1. Data summary
Toxic messages amount to 21% of the overall conversation touching on the COVID-19 pandemic and the role of the WHO in the crisis.8 Our analysis shows that 21 out of 100 tweets in our sample are expected to convey a rude, disrespectful, or unreasonable comment. During the time under study, the evolution of the toxicity in the conversations follows a slightly upwards trend, as shown in Figure 2. Baseline average toxicity increases from 26 March, when messages including a set of specific hashtags targeting the WHO gained popularity. The average percentage of toxic messages went from 18% to 25% after that date.
The maroon line on Figure 2 represents the daily percentage of toxic tweets as measured by computing the mean of the toxicity score of all tweets posted each day. Notably, surges in toxic conversation during the time studied correlate with a few external events. To identify those events, we extracted all tweets posted that day and the two following days. Then, we clustered them by combinations of hashtags and identified the most popular ones to determine the dominant topics around each peak.9
The main peak in toxicity, which took place around 29 March, was mainly related to two different conversations on the following topics: (1) an interview by the Hong Kong broadcaster RTHK with one of the WHO’s advisers touching on Taiwan membership, and (2) false information about Israel being removed from a map on the WHO’s website. The highest volume of toxic messages during the period studied can be traced back to those events. After that, toxicity levels surge again on 8 and 14 April, after the US president announced a review of funds to the WHO and eventually confirmed he was going to halt funding to the organisation.
We identify two additional media pieces that correlate with the highest surges in toxicity. First, a report by dailycaller.com titled ‘Top WHO official Tedros Adhanom Ghebreyesus Won Election with China’s Help. Now He’s Running Interference for China on Coronavirus’ (Hasson 2020), and also a piece by thegatewaypundit.com titled ‘Revealed: WHO Director General, Tedros Adhanom Ghebreyesus, reportedly ranking member of known terrorist organisation and China puppet’ (Hoft, 2020). Both Dailycaller.com and Thegatewaypundit.com are US digital-born outlets, and their audiences are respectively located at the right and extreme right of the ideological spectrum. As the analysis shows, their reports were central to toxic conversations that took place around 22 March and 2 April.
Figure 2. Expected percentage of toxic conversation across time
Note: Maroon line represents daily expected percentage of toxic tweets (mean of the toxicity score of all tweets posted each day). Dotted tuquoise line represents the percentage of messages including hashtags targeting the WHO (daily count of tweets including one of them, divided by total number of tweets that day). Vertical lines point to external events. Orange lines represent the WHO’s press conferences or meetings. Blue lines represent media coverage on three specific topics: WHO’s Director-General’s political background, an interview with a WHO representative questioned about Taiwan, and a report on links between the WHO and China. Finally, grey lines represent the US president announcing plans to review funding of the WHO and confirmation of ceasing funding.
At the end of March, a set of hashtags targeting the WHO quickly gained popularity; among them we found #WHOLiedPeopleDied, #WHOCriminals, #ArrestDrTedros, and #TedrosCriminal.10 To understand the contribution of these specific conversations to the overall toxic conversation, the dotted turquoise line on Figure 2 traces the percentage of messages including this specific set of hashtags. As shown, the percentage of tweets conveying toxic messages is significantly higher than that of the messages including the selected hashtags. Therefore, the toxicity in the overall conversation cannot solely be linked back to them. Consistently, further analysis is needed to understand the topics discussed in the toxic conversation, beyond these specific hashtags. Yet, interestingly, our analysis reveals that, on average, 33% of the total conversation including those hashtags was expected to be toxic, which represents a higher average toxicity than that of the overall conversation.
In order to understand the specific topics of the toxic conversations, we ran the following analyses. First, we identified all hashtags included in the tweets and ranked them according to the average toxicity score of the messages where they are included. Secondly, we extracted the URLs of all the messages and analysed the toxicity score associated with the ten most popular ones, as measured by the number of tweets including them. Finally, we analysed hashtags’ co-occurrence and identified additional topics with high levels of toxicity when two hashtags are included in the same text.
Figure 3 shows the results of the first analysis. For this, we focused on the second part of our period of study, after 26 March, when the baseline toxicity slowly grows across time, as shown in Figure 2. Before that date, toxicity is not clearly linked to a subset of hashtags to proxy the specific topics of those conversations. The purple line in Figure 3 represents the daily number of tweets that are expected to convey a toxic message. The dotted dark blue line represents the daily number of tweets including the top 22 most toxic hashtags.11
Figure 3. Volume of toxic conversation and hashtags across time
Note: Maroon line represents the daily number of tweets that are expected to convey a toxic message. Dotted blue line represents the daily count of tweets, including the top 22 most toxic hashtags. Bars represent the number of tweets including only the most popular toxic hashtags by reach. Some tweets include more than one of the toxic hashtags, as signalled by bars that run over the dashed blue line.
Overall, this figure shows the hashtags identified in our analysis are useful to understand specific topics in the toxic messages, especially those days when the blue and the maroon lines are about to overlap. Bars in this figure represent the total number of tweets, including only the most popular toxic hashtags by reach (n=11). As the height of the bars shows, those hashtags contribute the most to the toxic conversation. Figure 4 shows this contribution more specifically across time. Among these toxic hashtags, we find China (average toxicity score .40), the Chinese Communist Party (.30), and #chinesevirus (.41). Consistent with the previous results, and as indicated by the height of the bars, discussions including the hashtag Taiwan (average toxicity .30) and Trump (.31) were also prominent and spread across different days from late March.
Figure 4. Top toxic hashtags used across time
Note: Maroon line represents the daily number of tweets that are expected to convey a toxic message. Dotted blue line represents the daily count of tweets including the top 22 most toxic hashtags. Stacked bars represent the number of tweets including each of the most popular toxic hashtags. Selection of hashtags capped at 11 to improve visualisation.
Moreover, messages associated with the hashtags #WHOliedpeopledied (.33) and #Chinaliedpeopledied (.33) appeared frequently at different points in time.
Finally, among the most popular hashtags with the highest average toxicity score, we find two hashtags related to the Thai-speaking Twittersphere. One of these two hashtags touched generally on COVID-19 (โควิด19, 0.28 average toxicity) and the other one mentioned Vietnam specifically (เวียดนาม, 0.32).12 It is worth noting that the first COVID-19 case recorded outside China was in Thailand, which was also a priority destination for tourists from Wuhan during the lunar new year (see Figure 1). Also, Vietnam was among the first countries to be hit by the virus after China.
When looking at the combination of hashtags in the same tweet, we find that the co-occurrence of #China and #Taiwan triggers a surge in toxicity. More precisely, 91% of messages including both hashtags are expected to be toxic. It is worth noting that around 50% of tweets that include #ChinaLiedPeopleDied also include #WHOLiedPeopleDied, and 84% of tweets that include #biochemicalweapon in Chinese also include #newcoronavirus in this language. These figures give further information to identify the specific topics of the toxic conversations in our study and proxy the context where they took place too.
Finally, we identify the top URLs on tweets ranked by occurrence to better understand these conversations. Several of them are associated with tweets with very low probability on average of conveying toxic messages (Table 2). Yet a few of them are associated with higher toxicity scores on average than the baseline score of the overall sample (.21). This is especially the case of the URLs pointing to the tweet by US public broadcaster Voice of America, echoing the statement of the WHO’s Director-General with the following wording: ‘Politicizing the #coronavirus issue should be avoided by countries “if you don’t want to have many more body bags”, says @DrTedros’ (Herman 2020). Also, several URLs for Fox News get higher toxicity scores than the baseline of the sample. The URL with the highest score by this broadcaster points to a piece titled: ‘Coronavirus Coverup Underscores China’s Tight Grip on WHO, United Nations’ (Hollie 2020). The majority of URLs in this ranking are linked to US news media. Among the exceptions is a tweet by the WHO, and 29% of the messages including these tweets were expected to be toxic. The WHO tweet was posted on the official account of the organisation on 14 January, soon after the outbreak was identified in China, and included the following text: ‘Preliminary investigations conducted by the Chinese authorities have found no clear evidence of human-to-human transmission of the novel #coronavirus (2019-nCoV) identified in #Wuhan, #China’ (WHO 2020). Only six days after that, China confirmed there was evidence of human-to-human transmission in the COVID-19 outbreak (Wang and Moritsugu 2020).13
Table 2. Average toxicity associated with URLs
Given the relevance of some political leaders to COVID-19 and the WHO conversation, we have analysed the toxicity score associated with conversations mentioning a few of them.14 Strategically, we have selected the US, Brazilian, and Australian presidents. Among all of them, the US president is the most popular leader, as measured by the number of tweets. He is also associated with the highest average toxicity score (see Table 3). Around 30 out of 100 messages mentioning the US president are expected to convey a rude, disrespectful, or unreasonable comment. For comparison, Table 3 also included results relating to the WHO Director-General, who was most mentioned among those in the table. The average toxicity of messages mentioning him is lower than that associated with the US president, though.
Table 3. Toxicity associated with political leaders
Finally, we assess the status of the users that participated in the conversation on COVID-19 and the WHO from January to April 2020. In total, our sample includes 144,371 users and our results show that almost 7% of those were suspended (2%) or missing (4.7%) by the end of May. There is limited information available to know when and how a user is suspended. Twitter notes that accounts can be suspended when they are created from the same IP or linked to the same email; when they result from an automated sign-up process; or when they show an exceptionally high volume of tweeting with the same hashtag or the same username without a reply from that account (Roth and Harvey 2018).15 Its policy on platform manipulation also includes rules regarding aggressive, bulk, or deceitful activity. The platform takes action when multiple accounts operate to disrupt conversations or create artificial trends (Minshall 2020).16 Knowing why accounts are no longer active, i.e. missing, is even more complex. Yet, to identify the role of non-active users in the conversations about COVID-19 and the WHO, we classify them according to their use of hashtags with the highest toxicity scores on average. Table 4 shows that the highest percentage of non-active users are linked to #coronaviruschina and #US. Over 11% of users tweeting or retweeting messages including those hashtags were no longer active by the end of May. Following on this ranking, we find that, of users who posted messages including the hashtags #Chinaliedpeopledied and #WHOliedpeopledied, almost 11% and 10% respectively were also not active by May. Finally, with regard to the location of the non-active users, we find the majority of the first 50 users posting the hashtag #WHOLiedPeopleLied, associated with one of the highest toxicity scores on average, were related to the Indian Twittersphere.17 A heated debate took place in India after the WHO halted the hydroxychloroquine trial for coronavirus amid safety fears. The country manufactures 70% of the world supply for this drug (Sharma 2020; The Hindu 2020).
Table 4. Active and non-active users
Although our analysis identifies important peaks of toxic conversations around COVID-19 and the WHO, the majority of messages during the period studied were not toxic, even those including very abusive hashtags. On average, 21% of this conversation is expected to be toxic. Future research will have to determine whether this level of toxicity had any effect on public opinion and examine the causes of this incivility. With the evidence at hand, we can safely confirm that the toxicity patterns we identify are correlated with the increasing polarisation in the political and media sphere around the role of the WHO in the COVID-19 crisis. Consistently, the percentage of toxic tweets increases after 26 March (25%), when the effects of the pandemic worsened and the criticism from political elites intensified.
The authors thank the valuable work by Ariadna Net and Nathan Gallo as research assistants. Data used for this study have been partially collected by the CoMuNe Lab at Fondazione Bruno Kessler. The authors also thank the research team for their valuable input and suggestions, and the communications and administration teams for their support, at the Reuters Institute for the Study of Journalism. Finally, we thank Dr Subhayan Mukheerjee and Jayant Sriram, senior assistant editor of The Hindu, for providing us with context for this report.
1 Globally, Twitter has 166 million active users in 2020, almost 20% of them located in the US (Twitter 2020b). When it comes to specifically news about COVID-19, we know that in the early days of the outbreak, 30% of people in Spain said they used it, 18% in the US, and 19% in the UK, whereas only 6% in Germany (Nielsen et al. 2020).
2 List of related terms includes coronavirus, ncov, #Wuhan, COVID19, COVID-19, sarscov2, COVID. Further information on the selection criteria in Gallotti et al. 2020.
3 Previous literature has pointed to the lack of information on what and how much data one gets using the Twitter Streaming API, especially once the volume of the targeted conversation reaches the 1% threshold of the overall Twitter conversation (for an in-depth discussion see Morstatter et al. 2013). The dataset used for this study reached the 1% threshold at the end of February. A separate in-depth analysis based on the same data shows that the bias reported by Morstatter et al. 2013 does not significantly affect our results (see Gallotti et al. 2020: supp. fig. 1).
4 In this sample, there can be some spurious tweets containing the word who not necessarily intending to mention the WHO.
5 The toxicity model works directly in the original text in English, Spanish, French, German, Portuguese, and Italian. For all remaining tweets, the original messages were translated into English before computing toxicity scores. Translated messages amount to 18% of tweets in our dataset. As a robustness check, we compared the toxicity scores of the original texts to the translated ones. Our results show similar toxicity scores. More specifically, 21% of original tweets, in any of the above six languages, were expected to be toxic. The percentage was up to 23.5% when we translated them into English.
6 This tool has been specifically developed to give real-time feedback to moderators of newsrooms that manage audience comments. To evaluate its performance on our specific dataset, we manually classified two subsamples of 200 messages randomly obtained from our sample. Then, we compared the classification of the manual process, by two different coders, to those obtained with the trained model. The vast majority of the messages manually classified as toxic (85%) received scores higher than 0.4 by the trained model. The messages that human coders manually labelled as non-toxic received scores lower that. This indicates a reasonable agreement between manual and automatic judgement, and that the toxicity model was a robust way of generalising the analysis to the entire dataset. More information on the robustness check is available upon request.
7 Swearing or strong words have been replaced by [obscenity].
8 Hereafter for a subset of tweets, mean toxicity is equivalent to the expected percentage of toxic tweets, and the sum of toxicities is the expected number of toxic tweets in this set.
9 We used spectral clustering on an adjacency matrix of hashtag co-occurrence in studied tweets to understand the main topics of the conversations surrounding each peak.
10 Also #tedrosresign #tedrosliedpeopledied and different combinations of lower case and capital letters of the same hashtags.
11 To identify the most toxic hashtags, first we select those tweets that were on average in quantile 75% of toxicity (>0.28) and that have more than 0.5% of the total traffic (>1113 tweets). From that subset, we extract the hashtags and finally rank them by reach. In total we obtained 22 hashtags represented by the dotted dark blue line.
12 โควิด19 and เวียดนาม respectively mean COVID-19 and Vietnam in Thai.
13 See Figure 1 for more contextual information on the timeline of events.
14 Messages were filtered using the username and different combinations of their names with lower and upper cases. A separate analysis on Figure 4 shows a similar average toxicity score for messages mentioning the US president by using #Trump (.31).
15 For more information on the specific actions taken by Twitter during the COVID-19 pandemic see Conger 2020.
16 There are only hypothetical examples, not real ones, of tweets along those lines on the Twitter website. More generally, according to the platform 97 million accounts were challenged, which does not directly imply a suspension, over the first six months of 2019 (Minshall 2020).
17 To proxy their location we detect the language of the tweet among other criteria.
Published by the Reuters Institute for the Study of Journalism with the support of the Google News Initiative.
This report can be reproduced under the Creative Commons licence CC BY. For more information please go to this link.