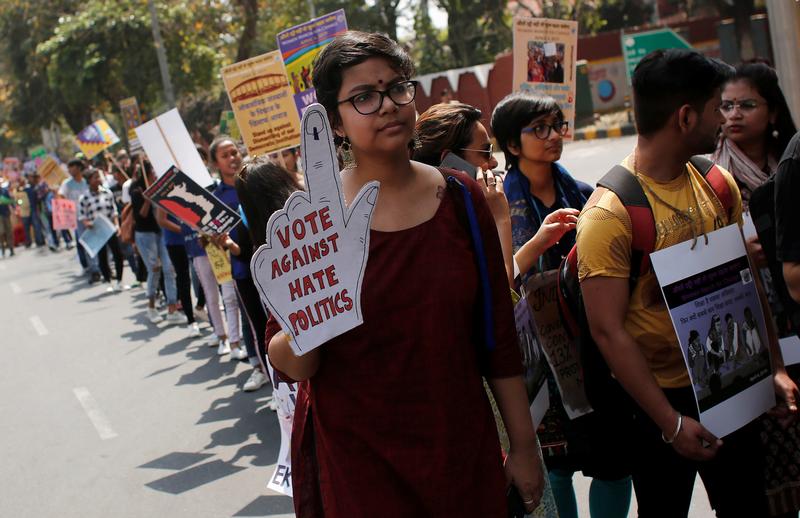
Indians take part in a protest march rejecting the current environment of hate and violence in the country, in New Delhi, India, April 4, 2019. REUTERS/Adnan Abidi
Indians take part in a protest march rejecting the current environment of hate and violence in the country, in New Delhi, India, April 4, 2019. REUTERS/Adnan Abidi
The National Crime Records Bureau (NCRB) in India, which has been tracking and publishing the country's crime statistics since the 1980s, stopped tracking religious killings and farmer suicides in 2017. This leaves no way of checking on whether either of these is trending upwards, although the frequency of reports in the media would suggest, anecdotally, that they are.
Similarly, there is no official tracking of hate crimes. An attempt was made in 2017 by Hindustan Times to start a hate tracker to document victims, but under government pressure the tracker was taken down. Editor Bobby Ghosh was asked to step down. A similar fate met the Hate Tracker created by online website IndiaSpend, and its editor Samar Halarnkar also resigned.
In my fellowship project at the Reuters Institute for the Study of Journalism, I worked under the guidance of communication researcher Dr Sílvia Majó-Vázquez to take in key lessons about how journalists can reliably step in to gather, clean and publish data when the government fails to do so.
First, I defined what would count as a hate crime: criminal acts committed with a bias motive in relation to a group characteristic of the victim such as race, ethnic background, religion, gender, physical or mental disability or sexual orientation. Then I scoured English language news media for reports of hate crimes between January 1, 2014 and December 31, 2020, copying links and noting details about each attack into a spreadsheet.
I excluded riots, because I did not have the time or resources to catalogue these fully. I excluded social media reports, because I didn’t have the resources to independently verify them. Finally, I excluded regional-language news outlets because I didn’t have the resources to accurately translate all of them.
The result is a Google Sheet with 212 incidents of hate crime reported in English-language media. Wherever possible, I have catalogued details such as the date, type of violence, gender, caste, and socio-economic details of victims and perpetrators, as well as their religion, politics, and the police response. I then analysed the data for patterns and trends. You can download the full report below for my findings.
In any database like this one – a database that relies on available sources, instead of sampling among all known occurrences – we cannot claim that the data is representative. The value of the cases I gathered speaks only to the characteristics of those that were recorded in English language media, and it also highlights the need to independently curate a comprehensive database for a better understanding of the prevalence and characteristics of hate crimes in India.
If you are planning to create your own database to plug a gap in official reporting, but don’t have the luxury of working with a communications researcher like Dr Majó-Vázquez, I have gathered some helpful lessons from my time working with her.
In the beginning, I was very keen on adding every little detail about each case. For example, when documenting the profession of the victim, I would specify whether the person was a meat seller, IT worker, a farmer or a student. This resulted in nearly 40 categories of work, which didn’t give a sense of the socio-economic class or motivations of the victim. With Dr Majó-Vázquez’s help, I narrowed down the professions of the victims to just five variables: blue collar worker, white collar worker, student, religious workers and other. This let me create a clearer picture of who was most likely to be targeted in a hate crime.
Your database could surprise you out of preconceived notions, but you cannot exclude or include data to confirm your own preconceptions without rendering your work useless.
It sounds like a small issue, but one or two typos can make a worksheet unsearchable. For example, under region, Uttar Pradesh spelled as “Utar Pradesh” meant that it would pull up as a different state while trying to filter search results. Copy editing is dull work, but it meant that I and others could easily analyse the data.
Initially, I had columns documenting ethnicity of victim, sexual orientation and the number of passive observers of the crime. In the case of the first field, the information became so repetitive as to be redundant. I realised that the nationality of the vast majority in the attacks was Indian, and that filtering for this information would not be of use. In the case of the second two fields, the information was available so rarely that the columns were no longer useful, because the available data would not be representative. Focus on the data that is available within your set.
While it makes sense in a database to refer to the Vishva Hindu Parishad as the VHP, I often had to explain my acronyms and shorthand to others. Use a separate sheet or the Comments tool to store footnotes about acronyms or links to explainers. This adds clarity so that anyone using the dataset will understand, without “breaking” your filters or cluttering your data set.
In a database, I realised some of the easiest searches happen with columns that are in the Yes/No format. For instance, did the police file a First Information Report (FIR, the equivalent of a police complaint): yes, or no? Did the police file an FIR against the victim or the perpetrator? By inputting the answers to such questions, I was able to see some unexpected results, for example: police filed FIRs against both the victim and perpetrator in 13% of cases.
Download Rachel’s full paper below to see all the trends and patterns her dataset revealed.