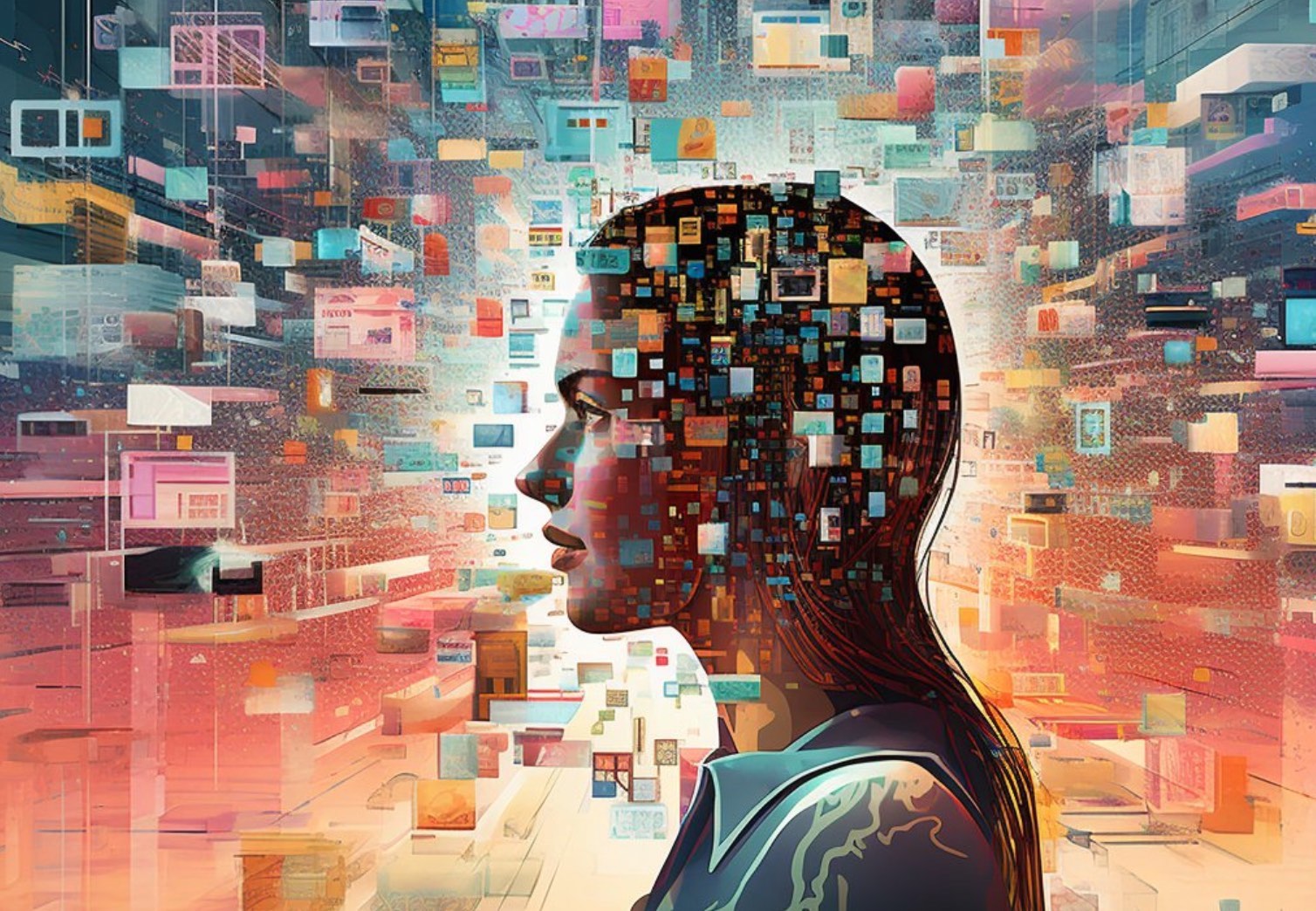
Illustration generated by the Midjourney 5.2 text-to-image model, using the prompt “An abstract image representing the uncertain future of digital journalism in the age of artificial intelligence.”
Illustration generated by the Midjourney 5.2 text-to-image model, using the prompt “An abstract image representing the uncertain future of digital journalism in the age of artificial intelligence.”
Innovation in journalism is back. Following a peak of activity in the mid 2010s, the idea of fundamentally reinventing how news might be produced and consumed had gradually become less fashionable, giving way to incrementalism, shallow rhetoric and in some cases even unapologetic ‘innovation exhaustion.’ No longer. The public release of ChatGPT in late November of 2022 demonstrated capabilities with such obvious and profound potential impact for journalism that AI-driven innovation is now the urgent focus of the senior leadership teams in almost every newsroom. The entire news industry is asking itself ‘what’s next’?
For many people in journalism the first half of 2023 was a time for asking questions and learning the basics of AI. What can ChatGPT actually do? What is generative AI? What is a language model? What is a ‘prompt’? How dependable are these tools? What kind of skills are required to use them? How fast is this technology improving? What are the risks? How much of all this is just hype?
Many newsrooms went further, providing their employees and audiences with statements or guidelines describing how they intended to approach the use of generative AI in their workflows and news products. Some even began publishing a few experimental articles written by ChatGPT. Very few, however, have yet taken specific steps to pragmatically and routinely apply these technologies in their newsrooms. Change is in the air, but specific initiatives are harder to find.
Over the past six months I have had the privilege of spending significant time discussing AI with the senior leadership of more than 40 news organisations, ranging from scrappy digitally-native newsrooms in Asia, the Middle East, and Latin America to many of the largest global news providers based in the US, UK, and Europe.
This access came as a result of my familiarity with AI-driven innovation in news, acquired from a combination of applied product innovation at large media companies in the US and UK, and from a small parallel academic career exploring the first principles of computation applied to news production and consumption.
My journey with many of the questions now facing news organisations began in Silicon Valley more than a decade ago, and my exploration of the GPT family of language models began in 2019 at the BBC. My career focus — a relative niche until the arrival of ChatGPT — was suddenly of great interest to many people in the news industry.
This article is based on a distillation of what I have learned from these conversations. It assumes a knowledge of generative AI’s general capabilities and potential, and examines some of the ways that large news organisations are thinking about its strategic and practical implications for their newsrooms. My intent here is to help advance the conversation beyond awareness and towards specific initiatives that can help move newsrooms forward in preparing for an uncertain AI-mediated future.
Any point of view regarding the application of AI to news is somewhat speculative, especially given the remarkable pace of advancement in AI functionality. Nonetheless, I believe that some clarity may be emerging.
Coming up with a list of things that your newsroom might be able to do using ChatGPT is fairly easy (Summarisation! Rewriting in a simpler style!). It is much harder to clearly identify exactly what it is that you are trying to achieve with generative AI, why you are trying to achieve it, how you might plausibly achieve it at scale in routine and professionally managed operations, and whether that achievement will even continue to be relevant as AI fundamentally alters the competitive landscape in the coming months and years.
To frame this discussion I will begin with an overview of potential strategies for using AI in news, before turning to options for the practical deployment of AI in newsrooms, and the infrastructural and organisational requirements needed to support those options. At the end of the piece I will offer a point of view for changes that we might anticipate for the news industry in the longer term.
Efficiency-focused strategies
The most obvious opportunities for applying generative AI to news are in bringing new efficiencies to specific and familiar steps within the existing news production workflows supporting an organisation’s existing news products. This ‘more-efficient-production-of-existing-products’ strategy is attractive in its simplicity, but its benefits will almost certainly be short-lived because it assumes that the existing media environment will continue in roughly its existing form.
It is increasingly possible that the competitive environment, product offerings, production workflows and business models of news organisations will change, possibly radically, as use of generative AI becomes ubiquitous and as AI-based media products appear.
There are early indications of this in the nascent generative search experiences offered by Google and Microsoft, in the user control of consumption experiences offered by well-funded news aggregators such as Artifact (launched by the founders of Instagram), in the declared intention to build a foundation language model around ‘X’ (or Twitter 2.0), and even in the early behaviour of tens of millions of early adopters of generative AI tools like ChatGPT and Midjourney, who have quickly learned how to use traditional media essentially as raw material for their own self-directed consumption experiences.
A ‘more-efficient-production-of-existing-products’ strategy is clearly a reasonable place to start, but it does not fundamentally compete with new AI-enabled experiences and therefore may not remain sufficient for long.
Product expansion strategies
A more ambitious AI strategy for news organisations lies in reimagining and expanding the scope and scale of an organisation’s news products in ways that only become feasible using generative AI. This ‘new-products-for-new-audiences’ strategy will likely be more enduring than an efficiency-only approach because it seeks to actively accommodate the expansion of audience choice that generative AI enables.
In essence this approach assumes that if generative AI is going to provide audiences with more control over their news consumption experiences (as pioneered by the Artifact app), then news organisations might as well offer that option directly. If news consumers can now control how they consume articles, then shouldn’t they be able to do that on a news publisher’s app or website?
The advantage of this strategy is that it offers a way to compete within an emerging AI-mediated news ecosystem while continuing to exploit the advantages that news organisations already possess — brand, trust in editorial processes, habit, etcetera.
Furthermore, that competition need not merely be aimed at existing targeted audiences but might now also be extended to entirely new audiences via new journalistic products that would have been editorially impossible without generative AI. Even small newsrooms now have the option of offering comprehensive multi-media news products to diverse audiences with radically different consumption behaviours and preferences.
Differentiation strategies
A more enduring strategy for news in the age of AI will necessarily be centred on differentiation and competitive advantage, offering exclusive news products that remain uniquely valuable to audiences even as the information ecosystem changes.
This ‘unique products’ strategy is challenging because it will be audiences that decide on the relative uniqueness of a newsroom’s products, not newsrooms. Pursuit of differentiation must therefore be clear-eyed, unsentimental and data-tested – magical thinking about vague specialness requiring little effort to implement is unlikely to be helpful here. A differentiation strategy might still potentially fit well with some of the values and brand attributes of traditional journalism, especially if the information ecosystem begins to significantly degrade under the onslaught of AI-generated content.
Some examples of familiar forms might include: proprietary news gathering using special access or special skills; audience trust gained from well-demonstrated verification and validation processes; richly contextual content produced from archives or from deep familiarity with subject matter; or perhaps a commitment to systematic coverage of particular subject domains using narrowly customised stories, such as local coverage or climate reporting.
All these opportunities, however, will likely need to be comprehensively optimised using generative AI to remain competitive within an AI-mediated information ecosystem, even if their core value is differentiated. Developing forms of differentiation will likely be very challenging for many news organisations, especially for those whose current product is largely built on packaging commodity information, however there may eventually be no alternative.
Techno-editorial strategies
A similar but even more ambitious potential strategy, probably available only to a few well-resourced news organisations or well-funded start-ups, is to seek to develop proprietary or competitively defensible information technologies and services centred on news – products that might resemble specialised intelligence tools more than traditional news products.
This option, which we might call a ‘techno-editorial product’ strategy, would likely reverse the relationship between the product and editorial functions that currently exists in most news organisations, requiring editorial operations to support product and business development rather than the other way around.
This kind of strategy might include provision of highly technical solutions such the systematic extraction of news events (and, critically, of news stories) from enormous streams of natural language text and speech, the creation and maintenance of proprietary datasets in story form, the systematic certification of that news, new tools for exploring and contextualising complex news, the extreme customization of news and of the experience of consuming news, etc.
Such processes and tools might resemble those used by government intelligence organisations, platforms like search engines, or processes and tools already offered by news organisations targeting financial professionals, but with much broader coverage and audience appeal.
Such a strategy would likely be research-led, would require substantial capital investment and needs to be justified by expected returns from high-value subscriptions. Such ‘techno-editorial’ businesses may not be well suited for reach-based news products but might nevertheless become significant components of an information ecosystem in which generative AI is ubiquitously deployed.
Training your own model
As news organisations look for strategic responses to generative AI, there are also a few options that are often discussed but which might be less attractive than they first seem. One of those ‘approach-with-caution’ strategies is that of pre-training a proprietary language model using a newsroom’s archive.
This is likely a bad idea for most news organisations for multiple reasons, including the difficulty in attracting the world-class machine learning talent needed to create a useful language model, the tiny size of the archives of even the largest news organisations relative to the training needs of a useful language model, and the substantial costs of periodic retraining, maintaining and operating a proprietary language model in the face of rapidly improving technology.
Furthermore there are few clear benefits available from training a proprietary model, even if it were competitive in performance to a commercial or open-source language model. Concerns about data security, vendor lock-in or a need for niche functionality can probably be addressed in much easier ways, including contractually, via abstraction of user interfaces, using model fine-tuning or using an open-source model.
A proprietary model built on the same transformer architecture as most current LLMs will still hallucinate, even if the entire training corpus is accurate, and a news organisation is unlikely to match the efforts underway by technology companies aimed at making language models more accurate. Training a proprietary language model may make sense for some very specific use cases in some news organisations with special information products (for example a ‘text-plus-financial-data’ multi-modal model at Bloomberg), but even in such cases a clear-eyed, careful consideration of the costs and benefits of such a strategy is essential.
Building a chatbot for archives
Another ‘approach-with-caution’ strategy that some news organisations have pursued or considered is that of building a proprietary chatbot that enables conversational, interactive access to the organisation’s current news and archive.
This option seems attractive given the success of ChatGPT. However, when considering a news chatbot, it is important to separately consider the interface component (i.e. interactive chat), the information component (e.g. news and its context) and the underlying technology used to deliver the experience (e.g. LLMs).
Despite the rapid adoption of ChatGPT, text or verbal chat as an interface is still very far from broadly accepted as a way of accessing any kind of information, much less news. Audience analytics for news bulletins built for voice agents like Amazon Alexa and Google Home have been disappointing, and early attempts at LLM-enabled chatbots by media companies have delivered little traffic.
This lack of success might be at least partly due to the relative inadequacy of news and news archives as a dataset powering such an interface, which for news organisations will be considerably smaller and more homogenous than the vast datasets that power ChatGPT or even search for that matter.
Furthermore, from a technology perspective it is very difficult to replicate the fluid nature of communication with an LLM using the techniques currently available for constructing chatbots from archives, which typically involve some kind of embedding-based search operation combined with interpretation of the results by the language model.
The result is often either far too many ‘I don’t know’ responses to specific queries, or references to ‘closest match’ archived articles in isolation from current context, an experience that can’t compete with internet-enabled chatbots like Bing Chat or Perplexity.ai.
Communication strategy
Regardless of where a news organisation might be in its path towards a strategy for responding to AI, there is an urgent strategic need that requires early attention – that of communicating the organisation’s approach to AI to stakeholders, funders, staff and audiences.
The urgency of strategic communication about AI for news organisations comes from the awareness that every individual connected with the organisation already has about the abilities of these tools and their potential for dramatic disruption. Most people in the news industry are already playing with the tools, reading the reports, assessing the potential and asking the obvious questions.
It would, of course, be ideal to patiently assess the situation, carefully devise a strategy, produce a plan for executing that strategy, and then communicate that strategy and plan – but that may not be possible to do quickly.
A more practical communication strategy at this stage might consist of acknowledging the situation, articulating how the organisation is engaging with and learning about AI, providing clear guidelines for its early or limited use, demonstrating new approaches to innovation, signalling adaptability and generally preparing for change. A tone of optimism and excitement for the potential of AI to help raise journalism above commodity information and to make it more accessible to many more people is also appropriate.
A strategic path to continued relevance
The common objective for almost all news organisations in navigating the coming AI-induced transformation is, bluntly, continued relevance.
News organisations, whether funded by ads, subscriptions, public funds or philanthropy, will seek to remain sufficiently valuable to enough people to ensure that those funding mechanisms continue to function.
The challenge of the coming transition to an AI-mediated information ecosystem is therefore to navigate a path that takes advantage of near-term opportunities for efficiency, medium-term opportunities for new products and services for audiences, and longer-term opportunities for re-imagining what news can become in a transformed information ecosystem.
This path obviously comes with considerable associated risk, specifically the risk of losing existing value and of not being able to develop new value to replace it, and may therefore require a greater tolerance of risk by leaders in news organisations. Developing that greater tolerance for risk, a tolerance perhaps closer to that of the technology companies that initiated this transition, might be the most important strategic step that a news organisation can take.
From strategy to projects
Any strategy for adapting a newsroom to an information ecosystem defined by generative AI is of little use without specific, practical projects that translate that strategy into useful outcomes.
Identifying such projects is obviously difficult during this current period of exceptionally rapid change, because of the considerable risks of wasted investments, embarrassing missteps or potential damage to brands or trust. In addition, projects can only contribute meaningfully towards a strategy if they can be applied in routine, day-to-day operations, rather than merely as testable prototypes or demonstrators.
To move forward, therefore, we need to identify categories of projects that might advance newsrooms towards an AI-ready future while minimising risks, and also identify the infrastructure requirements needed to deploy those projects routinely at scale when that time comes.
Back-end projects
A relatively low risk category of generative AI projects is purely back-end applications. These are applications with no direct audience-facing output, not even draft text, but which instead deliver their value to journalists or to businesses. These can include efficiency-focused or quality-focused tasks such as tagging, other kinds of categorisation, headline and SEO suggestions, assembly of newsletters from pre-existing copy, copy-editing, brainstorming and ideation, early research, some analysis, etcetera.
In addition to being relatively low risk, back-end AI applications are also relatively easy to implement as they are often ‘loosely coupled’ to news production workflow and infrastructure. Such applications can sometimes be managed by stand-alone tools disconnected from the primary publishing stack of the organisation, operated by specialised staff separate from the main editorial workflow.
Language task projects
A more ambitious but still relatively low risk category of generative AI projects include those applications that produce draft text by modifying source text in some way. These applications use language models solely for ‘language tasks’ and should not introduce any information content into the draft that was not already present in the source document.
They explicitly do not depend on the knowledge available to the model as a result of its training data, and they therefore reduce (but don’t eliminate) the risks of hallucinations, biases and other issues.
Examples of language tasks include summarisation, simplification, stylistic re-versioning, rewriting text for particular channels (social media, topic bulletins, etcetera), script-writing for audio or video, translation...
Language tasks can be done using any form of model access, such as the ChatGPT user interface, custom-built API-driven tools or even as new features integrated into content authoring and management systems. Language tasks can also be part of any strategy, including strategies based on efficiency, on new products or on differentiation. They are a fundamental category of journalistic task in an AI-enabled newsroom.
Knowledge task projects
A higher risk category of generative AI projects are applications that produce draft text with information content that originates in the language model itself, rather than from a source document.
These applications perform so-called ‘knowledge tasks’, because they are doing more than merely modifying language – they are true ‘authoring’ applications of language models.
The increased risk associated with knowledge task projects comes from the significant potential for hallucinations, simple error from training data, biases, out-of-date context and other limitations inherent in language models.
Nonetheless, if these risks can be managed, knowledge tasks offer a substantial range of news products including provision of context for stories, explainer-like background content, different interpretations of events based on historical context, and even full articles, especially on evergreen, commodity subject matter.
Mitigating the risks associated with knowledge tasks requires editing processes designed to detect error and inappropriate content – non-trivial tasks, as evidenced by the difficulties experienced by CNET in publishing knowledge-based content from language models. Nonetheless, there are clearly many significant journalistic opportunities available from knowledge tasks, and these opportunities will likely increase as we collectively learn more about how to manage and edit their output and as we begin to use AI to help do that.
As with language tasks, knowledge tasks can be integrated into workflows in different ways and can contribute to different strategies. They too are a fundamental category of journalistic task in an AI-enabled newsroom.
Medium-to-medium transformation projects
A particularly ambitious category of generative AI projects for news are applications that transform information content from one information medium into another, for example from text into audio, from text into video or from text into graphical images.
Unlike language tasks and knowledge tasks, these applications typically depend on special-purpose medium-to-medium transformation models, often used in combination with general-purpose large language models within complex workflows.
Such special-purpose models include speech-to-text models (transcription), text-to-speech models (synthetic voices), text-to-video models (synthetic avatars, automated generation of B-roll video, etcetera), text-to-image models and others. These tools are still at an early stage. But they are developing very quickly, are widely available, and already easily match human quality in many cases.
Furthermore, the potential of this category of applications is likely to increase with the imminent arrival of so-called ‘multi-modal’ functionality enabling richly descriptive image-to-text, video-to-text and other transformations.
Examples of potentially high-value journalistic tasks that can be accomplished using these cross-media models include the automated or semi-automated creation of text articles from audio or video source material, the creation of audio and video news products from text articles, the transformation of text articles into graphical stories or videos, and the automated or semi-automated creation of podcasts from articles.
These tasks are most useful for a product expansion strategy. They can often be achieved using just model vendor user interfaces and so have the potential to enable relatively low-resource newsrooms to quickly offer multimedia content at significant scale.
Some potential barriers to implementing this category of projects include the need for an editorial producer with experience in the output medium to ensure quality, the not insignificant cost of using the specialised models, and the challenge of distributing the same story in several different media.
Listening and monitoring projects
The project categories described above all focus on novel ways of producing news products using AI, but practical projects focused on news-gathering are also viable using large language models.
The term ‘generative AI’ has an obvious built-in bias towards the generation of media, but large language models and multi-modal models can also read, listen and soon observe at enormous scale, enabling entirely new ways of reporting news.
The kinds of news-gathering tasks that these models can perform extend far beyond the earlier generation of ‘social listening’ tools, which usually just searched for keywords in the feeds of social media platforms. The ‘natural language understanding’ (NLU) abilities of LLMs can not only read, but interpret, evaluate, analyse, synthesise and summarise.
Furthermore, they can do this not just with natural language text and speech, but also with structured data — as clearly demonstrated by OpenAI’s new ‘code interpreter’ add-on to their GPT-4 LLM, and likely soon with visual information in images and video. Projects based on NLU and ‘reporting at scale’ are already underway at newsrooms, including small newsrooms. Such projects may be most appropriate for a differentiation strategy and might enable newsrooms to build out and defend special abilities to report systematically on specific domains.
Advanced projects
Our understanding of potential applications of AI in news is still nascent, not only because of the rapid development of new functionality but also because we have just begun to explore the potential of these tools for news work.
The projects described above are easily possible right now, with existing functionality, but there are also several ‘near frontiers’ of functionality that will likely open up significant new potential. One of these is the advent of multi-modal functionality, which is already available in limited forms in Bing Chat, MidJourney and soon GPT-4, and is likely to become increasingly powerful for applications that cross between language and visual information.
A second near frontier is the advent of LLM ‘agents’, pioneered by small examples like AutoGPT and BabyAGI. These approaches use LLMs to deconstruct high-level tasks expressed in vague terms into small, specific, actionable tasks that are then carried out by the LLM. They offer intriguing possibilities for automating some investigative journalism, and for scaling investigative reporting into an ongoing and systematic function.
The AI strategies and specific AI projects described here illustrate that newsrooms have tangible and specific options for moving forward towards an AI-mediated information ecosystem, but these strategies and projects are insufficient. Producing professional AI-enabled news products, using AI-enabled workflows, at sufficient scale to make a difference, day-after-day and month-after-month, requires something more. It requires infrastructure.
Old-school AI infrastructure
In the decade prior to the rise of large language models, infrastructure for AI in news organisations meant something different than it does today. It meant data warehouses and data lakes, a well-structured and well-maintained metadata schema, libraries of embeddings, an expensive data science team, a large monthly AWS bill and a product roadmap focused on training small, specialised ‘machine learning’ models from scratch using small volumes of proprietary data.
These roadmaps often included business-focused models predicting propensity to subscribe, propensity to churn or willingness-to-pay, journalist-focused models enabling a host of special functions useful for a handful of special stories, and audience-focused models such as semantic search and, of course, different kinds of recommender systems.
This kind of AI infrastructure is still very valuable and useful, even if affordable only by a small number of elite news organisations, but it is very different from the infrastructure required to apply generative AI.
Professionalised prompt management
A fundamental and permanent requirement for applying generative AI to news work is infrastructure that enables the professional development, testing and deployment of prompts.
AI models will be a permanent part of the future of news, and controlling those models in the service of useful journalistic work will be a central function of editorial organisations.
That control will be exercised through prompts. Whether back-end tasks, language tasks or knowledge tasks, and whether employed as part of an efficiency strategy, a product expansion strategy or a product differentiation strategy, all applications of generative AI in newsrooms are fundamentally dependent not just on the models used to execute them, but also on the prompts used to direct those models.
Permanent, professional mastery of prompts does not look like journalists casually cutting-and-pasting from a dozen options in a Google Doc into ChatGPT, but instead looks like a professional ‘prompt-to-publish’ pipeline that enables systematic and quality-controlled management of every aspect of prompting, outputs, editing and deployment.
This includes prompt design, assembly, evaluation and testing, ‘certification’, metadata, storage and retrieval, versioning, iterative improvement, usage tracking, analytics, output editing, training and more. Even just prompt design can involve explicit task definition, application of system roles and ‘custom instructions’, development of few-shot examples, management of context size, prompt templating, multi-prompt staging and more.
Just evaluating the outputs of prompts applied to stochastic models fed by diverse source documents presents a combinatorial editing challenge unlike anything previously seen in news work. All of this requires infrastructure – databases, tools, user interfaces, schemas, integration, processes, analytics, training and documentation.
Interfaces between prompts and journalistic tasks
Assuming that adequate ‘prompt-to-publish’ infrastructure is in place, a newsroom still requires an interface between this infrastructure and its journalists.
For many journalists this is unlikely to be the raw prompt, which for useful tasks will likely be long, quite complex and probably ‘certified’ as tested and reliable according to some accepted quality control process. Instead most journalists will likely access prompts via buttons and controls that produce draft outputs for editing and refinement.
Such interface infrastructure could consist of either stand-alone journalistic ‘co-pilot’ tools with separate experiences for prompt management and prompt use – something already seen in Google’s ‘Genesis’ interface, in various ‘News AI’ tools from start-ups and in some in-house tools developed by newsrooms. It will also require a comprehensive integration into an organisation’s existing content management infrastructure or possibly even as an entirely new form of CMS designed specifically for AI workflows.
Maintaining control of these tools, of the buttons and functions they provide, and of the prompts behind those buttons will likely be critical for journalistic independence in an AI-mediated news ecosystem.
Infrastructure for personalised experiences
Even with a coherent strategy, a set of applications that support that strategy and a prompt management process that professionalises the execution of those applications, the extent of AI-enabled news production will still be limited by the available content management, serving and distribution infrastructure.
This is especially true of a product expansion strategy executed using language tasks or medium-to-medium transformations.
Producing 5, 10 or 20 different variants of every story, suitable for a wide variety of audiences, situations and consumption contexts, might be far easier than actually serving each of those variants to the right user at the right time. This is a personalisation challenge, but one that is quite different from the usual interpretation of ‘personalisation’ in most newsrooms – which tends to focus almost exclusively on personalised recommendations of one-size-fits-all content artefacts.
This new requirement is, instead, for personalisation of the story experience, and it therefore requires infrastructure that can store, select and serve different variants of a story to different users or different user segments in different situations – in addition to continuing to recommend relevant stories.
A simple form of experience personalisation, being pursued by several newsrooms, is to set up a separate channel or even an entirely separate brand from which to serve a newsroom’s stories in new AI-generated formats.
A more advanced form is to offer direct user control over their consumption experience, for example in the way that the Artifact news app offers article consumption as summaries, simplified text, emoji stories, poems or as audio readings by celebrities (see illustration above).
A more complex form of experience personalisation is to automatically adapt a user’s experience using behavioural data and contextual signals.
Each of these approaches can open up access to a newsroom’s journalism to more people, but each also requires infrastructure that can support the management and serving of story variants.
News-gathering infrastructure
Infrastructure is also required to take advantage of opportunities to use LLMs to substantially scale news-gathering by monitoring and analysing large volumes of source documents – perhaps as part of a differentiation strategy focused on competitively defensible coverage of a particular news domain.
A starting point is infrastructure that enables constant access to source material, which might exist in different text formats, or in audio or video form that must first be converted to text transcripts using AI speech-to-text tools, or possibly even in large datasets that are frequently updated. Such ‘monitoring’ infrastructure might range from a simple list of sources to a complex web crawler that continually ‘reads’ portions of the internet to maintain an awareness of domain events.
The monitoring that such infrastructure performs will depend on a system of prompts and a system that manages the summaries and assessments that are the outputs of such prompts.
This all requires databases, data schemas, access tools and filtering functionality. Such functionality can be built at a small scale and used as a supplement to a manual monitoring workflow, and several newsrooms are already doing so.
Infrastructure flexibility
Despite our efforts to identify a useful way forward amid the uncertainty of the present moment, there remains a real possibility that AI might enable an entirely new interface to journalism, delivering experiences of news that are not centred on discrete text, audio or video artefacts.
We see early signs of this in the form of chat interfaces, generative search and early conversational voice interfaces enabled by language models. Despite the caution provided above regarding an archive chatbot strategy, and despite evidence from audience research indicating a preference for passive rather than active news consumption, it is still possible that we may soon be interacting with news in entirely new ways.
Given this uncertainty, it may be useful for news organisations to reexamine their technical architectures and infrastructure strategy from the perspective of an increasing need for flexibility. This might involve reexamining build-vs-buy decisions, refactoring brittle, tightly coupled architectures, or even attempting to identify various possible product scenarios and a path to infrastructure that could support those scenarios if they develop.
Any such reevaluation should consider the many new options for AI-enabled software architectures and AI-enabled software engineering that are appearing. The increasing need for flexible infrastructure is accompanied by new AI-enabled techniques that may make it easier to design, build and maintain such infrastructure.
News organisations will need to operate differently
A news organisation with a coherent, well-articulated strategy for responding to generative AI, delivered via a portfolio of well-conceived applications supported by a professionalised prompt management process and delivered to audiences via a personalised publishing stack would clearly be well-placed to adapt to an AI-mediated information environment.
But such an organisation would also likely need to operate differently from a traditional digital news publisher, and its organisational structure would probably need to change substantially in order to support those differences. Furthermore, the skills and talent required to operate successfully in this environment are likely to also be different.
Discussions of likely changes in the structure of news organisations often focus on the potential for AI to either replace or augment traditional jobs. But the reality is likely to be more complicated than that.
It is quite certain that many newsroom tasks will be either replaced or made moot by AI, but it is also certain that many new tasks will appear. It is obviously difficult at this stage to predict how old and new tasks will be assembled into individual jobs, teams and department-level functions, and different newsrooms with different strategies will clearly do this differently. Nonetheless we can make some informed speculation using the likely drivers of organisation change as a starting point.
Drivers of organisational change
If we consider what generative AI can do, how it is being used for news applications in a nascent way and how news organisations talk about it, then we can make some plausible assumptions about how news organisations might change in response to it:
The collective organisational influence of all these assumptions can perhaps be summed up in a single word: autonomy. Those teams that adopt and master AI will be able to do much more, with many fewer dependencies on other parts of the organisation.
An AI-native news organisation
The enablement of increased autonomy by AI suggests to me that the productive units of AI-native news organisations might be small, AI-empowered, multi-disciplinary and self-directing teams operating relatively independently from each other and each focused on serving a specific audience or audience need.
In this scenario the organisation itself becomes somewhat federalised, providing an environment within which self-directing teams can be productive and impactful but not directing their work.
This federal organisation provides brand, values, certification of quality, monetisation, financial stability, enabling infrastructure, training and, of course, general strategy. The atomic teams provide adaptability, fast decision making, audience and competitive awareness and, of course, routine production of valued content via AI-augmented workflows.
Implementing such a federalised organisational structure would clearly be challenging for many news organisations, not least because of that eternal and vaguely-defined bugbear of newsroom change management: culture.
A practical, near-term response being explored by some pioneering newsrooms is the possibility of becoming a ‘two-speed organisation’, taking advantage of the new autonomy available from AI to set up teams that are loosely coupled to existing workflows. A longer-term response, already underway at several newsrooms, is to re-evaluate hiring and performance criteria to emphasise the skills and talents needed to form a more autonomous AI-empowered culture.
Skills and talent
The most valued skill in an AI-empowered news organisation will likely be the same as it has been in traditionally configured news organisations.
Editorial judgement – the ability to maintain a keen awareness of the deep informational needs of an audience or society, identify stories that meet those deep needs, verify and contextualise those stories, and then communicate them to audiences in clear and engaging forms – will probably remain the foundation of journalism.
How editorial judgement is exercised, however, may change in ways that require substantial new skills and different talents. These might include an ability to work with abstractions and systems, to analytically understand audiences and their needs, to engage with complexity, and to remain curious and to learn continually.
Organisations will obviously need to identify, support, incentivise and retain their most innovative and adaptable employees, but they will also need to supply those AI-empowered employees with leadership that is perhaps more entrepreneurial, more skilled at motivating and coaching, and perhaps less managerial or political.
Specific technical skills with specific AI tools or models may, surprisingly, be less important due to the near-universal accessibility of the interfaces. Merely operating AI will likely be much easier and therefore less valuable than wielding it skilfully as a genuine superpower.
The AI moment
The development of generative AI has placed journalism at the cusp of significant change, variously equated to the iPhone moment, the birth of the internet and even the appearance of the printing press.
The significance of the moment has been understood and appreciated by the senior leadership of most newsrooms, and many are already moving forward towards specific initiatives and experiments aimed at preparing for an AI-mediated future.
Considerable attention is rightly being given to potential harms, to the ethics of using AI in journalism, to influencing regulation and legislation, to the potential for AI-created misinformation and disinformation, to education of audiences and ‘AI literacy’ and to the development of early guidelines to orient news organisations as they begin this new transformation of their industry.
This attention is necessary and valuable, but it is not enough. Journalism must also engage with these new tools, explore them and their potential, and learn how to pragmatically apply them in creating and delivering value to audiences.
There are no best practices, textbooks or shortcuts for this yet, only engaging, doing and learning until a viable way forward appears. Caution is advisable, but waiting for complete clarity is not. So-called ‘second mover advantage’ is only available to those who are well-prepared to move when the time comes.
The next information ecosystem
Looking further ahead, the need for hands-on familiarity with applied AI in journalism becomes even more critical because of the likelihood that the entire information ecosystem within which journalism exists will undergo transformation.
What will journalism look like, for example, in an environment in which text, audio and video is fluid and malleable to the preferences of each individual consumer? What should the tangible output of a newsroom be in an environment in which that output is consumed primarily by machines? How will a coherent record of news – an archived ‘first draft of history’ – be maintained in such an environment? What might news become when useful reporting can be done on almost every word of text or speech, or every byte of data, produced in public by society? How will newsrooms capture value from their work in such an environment? What will that work be?
These questions, and others that are similarly fundamental, may not become relevant for years, or even decades, but merely participating meaningfully in that discussion will require newsrooms to possess far more tangible expertise of AI-augmented journalism than any now possess.
Progress in the face of uncertainty depends on developing and maintaining options, but options require situational awareness, and situational awareness comes from authentic engagement with the environment. For journalism that environment will almost certainly be shaped by AI.
In this article I have attempted to describe a few ways in which news organisations might build on the awareness of AI that they have developed since the launch of ChatGPT with specific strategies, projects, infrastructure and organisational changes.
These suggestions are my own interpretation of what I am observing and hearing, and they are by no means exhaustive or complete. It would be reasonable to expect people who staff or lead news organisations to exhibit frustration or even resentment at the prospect of even more impending change, but this is not what I have observed.
Instead the predominant tone of my conversations about AI has been one of optimism and excitement, largely at the opportunity presented by AI to further realise an ideal of journalism that motivates many of us who work in and around newsrooms. If you could bring that ideal to life, without regard for the scarcity of resources, where would you start?
David Caswell is a consultant, builder and researcher focused on AI in news. David has led news product innovation at the BBC, Tribune Publishing & Yahoo! and publish peer-reviewed work. He is a regular speaker of our newsroom leadership programmes. This piece, which was written entirely by hand and without the use of AI, was first published here.
At every email we send you'll find original reporting, evidence-based insights, online seminars and readings curated from 100s sources - all in 5 minutes.